Introduction: Balancing Data Utilization and Privacy in Healthcare
The healthcare industry stands at a crossroads where the utilization of patient data for advanced medical research and treatment must be balanced with the imperative of preserving privacy. The incorporation of privacy-preserving technologies (PPT) is critical in achieving this balance, ensuring that patient data is used responsibly and securely. Healthcare organizations should employ a holistic privacy strategy to ensure that their patient data is kept private and secure end-to-end for all possible computations and use-cases.
The Challenge: Data Privacy in a Digital Age
The digitalization of health records and the rise of telemedicine have heightened the risks of data breaches and unauthorized access to sensitive patient information. These challenges necessitate robust solutions that protect patient data while enabling medical advancements.
A Holistic Privacy Strategy: Selecting Multiple PP-Techniques for Different Purposes
The combination and integration of differential privacy with federated learning, Fully Homomorphic Encryption (FHE), and Multi-Party Computation (MPC) offers a robust strategy for secure data handling in healthcare:
- Federated Learning for Collaborative AI Training: This approach allows hospitals to develop AI models, such as predictive algorithms for chronic diseases, without sharing raw data. It ensures that sensitive information remains within the hospital’s secure environment.
- FHE for Secure Data Processing: FHE enables hospitals to perform analyses and make predictions on encrypted data. For example, using encrypted patient data to predict treatment outcomes without ever decrypting the information.
- MPC for Collective Insights: MPC facilitates the secure pooling of data insights from multiple hospitals. This enhances the understanding of widespread health trends while keeping individual patient data private.
- Differential Privacy for Additional Security: Integrating differential privacy into this framework adds an extra layer of security. It ensures that the data shared or analyzed cannot be traced back to individual patients, even in aggregated reports or AI model training. This is particularly useful in research involving rare diseases or unique treatment responses, where individual patient data might otherwise be identifiable.
Case Study: Personalized Cancer Treatment
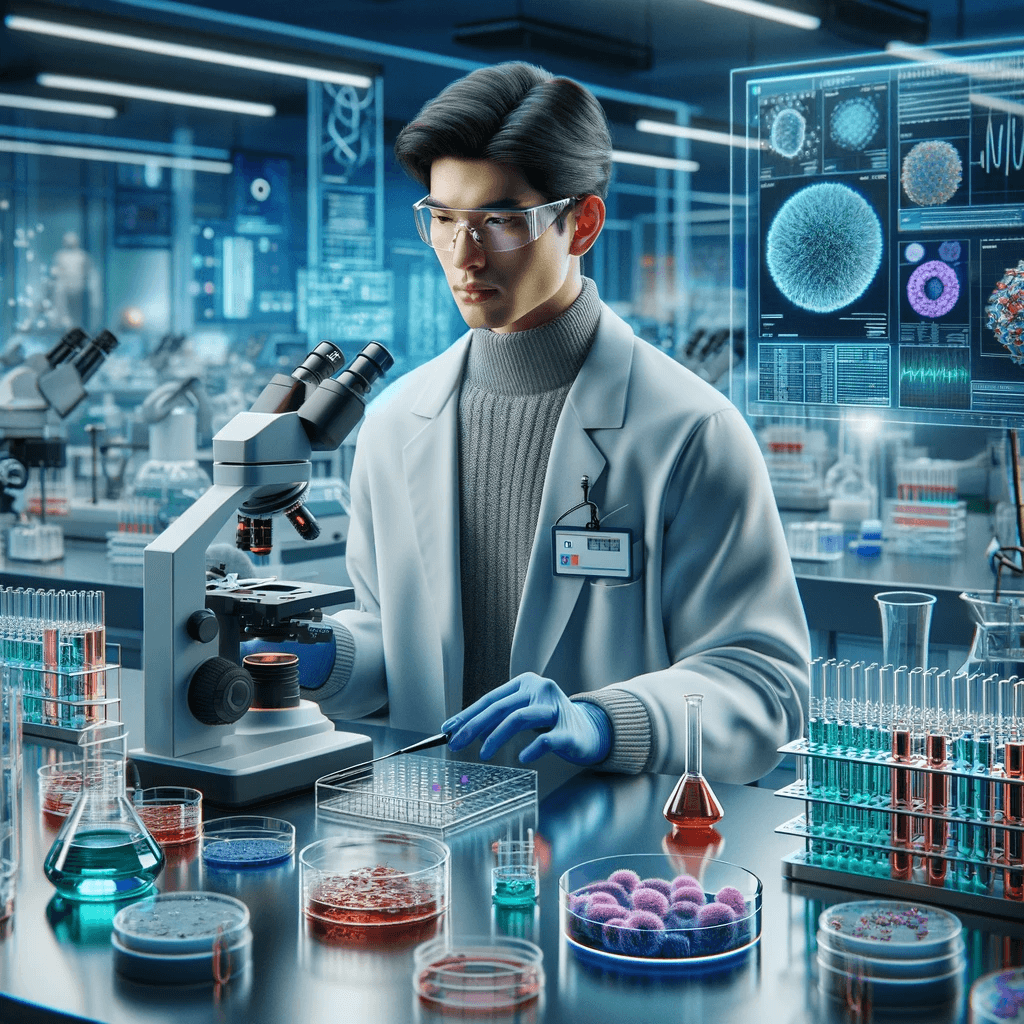
To illustrate the efficacy of these PPTs, consider the scenario of personalized cancer treatment:
- Situation: A network of oncology centers seeks to develop an AI model that can predict the most effective treatment plans for various types of cancer, based on genetic markers, treatment histories, and patient outcomes.
- Application of Federated Learning: Each center trains the AI model on its own patient data. The model learns from a diverse set of data without the data ever leaving the individual hospitals, thus preserving patient privacy.
- Use of FHE in Diagnosis and Treatment Planning: Once the model is trained, a new patient’s data is encrypted and sent to the cloud for analysis. The FHE allows the model to make predictions (e.g., which chemotherapy drug would be most effective) on this encrypted data, without ever decrypting it, thereby safeguarding the patient’s sensitive information.
- MPC for Collective Learning: The participating centers use MPC to securely share insights gained from the AI model’s predictions. This process allows for the refinement of the AI model and the generation of broader insights into cancer treatment efficacy without compromising individual patient data.
- Incorporation of Differential Privacy: When sharing results for broader research purposes, differential privacy is applied to ensure that the data cannot be traced back to any individual patient. This is crucial in cancer research where patient data might include rare genetic markers.
Integrating HintSight’s Advanced FHE Technology
HintSight’s technology, with its novel approach to FHE, can significantly enhance this framework. By enabling faster processing of encrypted data, HintSight’s solution makes real-time, secure data analysis feasible. This technology is particularly valuable in situations where rapid decision-making is crucial, such as adjusting cancer treatment plans based on real-time patient responses.
Conclusion: Pioneering a Privacy-Centric Approach in Healthcare
The integration of federated learning, FHE, MPC, and differential privacy represents a significant step forward in protecting patient data in healthcare. This multi-faceted approach not only ensures the privacy and security of sensitive patient information but also facilitates the advancement of personalized medicine. HintSight provides technologies are integral to this paradigm shift, offering efficient and secure solutions that uphold the sanctity of patient data while enabling groundbreaking medical research and treatment.